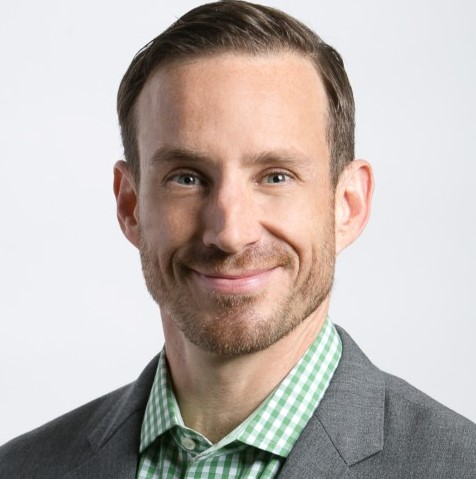
The COVID-19 pandemic shined a spotlight on the urgent need to modernize the nation’s public health system. Despite success in rapidly developing vaccines, the unprecedented public health emergency also exposed significant gaps in U.S. public health infectious disease data collection and analysis methods which are critical for identifying behavioral risk factors and preventive actions.
The Problem
Unfortunately, inefficiency remains a hallmark of the U.S. public health surveillance system due to the following two lingering issues:
- Disparate data collection systems
The CDC receives data from all 50 states and more than 3,000 local jurisdictions and territories. Hospitals, providers, and laboratories use a variety of systems to collect this data which is then reported to state, city, and local public health agencies. The information is then shared with CDC and other federal agencies. In general, each city, county, and state decide what information is collected, as well as how and when it can be shared with CDC.
What’s more, many current systems rely on disease-specific monitoring and manual data entry, which substantially burdens federal data partners. State and local reports to CDC are often delayed because the systems and data are simply not interoperable.
- Antiquated data-sharing methods
While data is increasingly shared via automated, electronic exchanges, some data is still being sent by fax machines, excel spreadsheets, or even by phone. The CDC encourages standardization, but it lacks the authority to receive data directly without establishing a data use agreement with each state and local jurisdiction.
As a result, the agency must manually clean the data before conducting the analyses needed to provide an aggregated picture of public health. It can take weeks or even months to share the data with public health authorities, providers, and the scientific community,
The key challenge: how to collect and share information more efficiently so that information turns into actionable insights that can shape important public health decisions?
The Progress
The good news is CDC is leading multiple initiatives to make our public health infrastructure more connected and resilient. The CDC’s Data Modernization Initiative (DMI), launched in 2020, is a multi-year, billion-dollar-plus program to modernize core data monitoring and surveillance infrastructure across the public health ecosystem with the goal of enabling faster, actionable insights to support better decision-making. The recently created Office of Public Health Data, Surveillance and Technology will support this effort.
Four key actions for fully modernizing the public health data infrastructure, and expanding data collection and sharing are:
- Adopt a Scalable, Federated Data Mesh Infrastructure
Today’s network of siloed, disease-specific systems creates significant redundancies and inefficiencies. It cannot scale to support the level of data aggregation, access, and speed public health agencies need.
A scalable, federated data mesh infrastructure would allow federal agencies to curate high volumes of rich, interoperable data across their ecosystems. They could then accelerate their aggregation and analysis, and in turn, their public warnings and outreach, which are critical for fast-moving threats such as infectious diseases.
By decentralizing data repositories, a data mesh allows those who are most knowledgeable about their data to control it, namely the public health entities functioning as nodes in a network. Via the mesh, the CDC would engage with electronic health records (EHRs), lab reports, genomic sequencing information, immunization, and other records. State and local agencies would then similarly engage. With CDC defining mesh policies and managing the mesh, data can be ingested, cleaned, standardized, and provisioned for use.
With such a decentralized information technology architecture, federal agencies could also integrate technology to facilitate HIPAA-compliant patient record matching. This could be achieved without creating bottlenecks typically associated with centralized reporting and dissemination.
Powered by robust metadata, search features and a centralized data catalog, the mesh would enable authorized personnel to effectively find, access, aggregate, and analyze public health data. This information could also be merged to support the principal guidelines for sharing and managing data adopted by research institutions worldwide, known as the FAIR Principles (Findable, Accessible, Interoperable and Reusable).
- Protect Privacy
Protecting the confidentiality of patient health information must be a top priority when modernizing public health infrastructure. The data mesh described above can integrate privacy-preserving record linkage (PPRL) technology which allows for data to be linked across different data sets without exposing individuals’ personal information.
PPRL technology maintains HIPAA compliance while enabling the matching of identifiable patient data without compromising patient privacy and confidentiality. For example, PPRL employs hashing to convert variables such as names, birthdates, and addresses into encrypted tokens that preserve the original values.
Linking data at the patient level enables a comprehensive view of an individual’s health, allowing researchers to answer questions that would otherwise require extensive primary data collection or complex data use agreements.
By integrating PPRL with standardized Fast Healthcare Interoperability Resources (FHIR) data components, public health agencies would be able to ingest and collect data from multiple sources and feed it into scalable analytics and modeling tools.
- Expand Data Sources
Currently, limited EHR and social determinants of health data (such as access to transportation, rates of chronic disease, food insecurity, and crime) are interoperable via the established standard – the United States Core Data for Interoperability (USCDI). This data should be augmented by structured health data which is currently siloed in other agency systems including:
- Geospatial data such as walkability and access to care
- Remote-sensing data, such as wastewater testing and satellite imagery
- Mobility data from smartphones, GPS, and sensors along highways
By layering additional data from siloed health systems and non-health sources, public health agencies could enrich the baseline USCDI data to gain deep insights. Recent efforts demonstrate the value of multilayered data to track the spread of COVID-19 in wastewater samples across the country, understand the impact of social distancing during the pandemic, and predict obesity rates.
While encouraging, however, these results are limited in scope. Real-time, actionable surveillance at scale is impossible because of the lack of interoperability across data sources. Alternate approaches that bring more data into public health models and simulations must be pursued.
By extending interoperability and connecting the universe of rich, relevant data, public health agencies could boost the accuracy of prevalence estimates, counter-balance biases in traditional data collection, effectively target control and prevention strategies, and better allocate resources.
- Harness Intelligent Automation
Modernizing surveillance systems without burdening the public health workforce is a major challenge.
Public health agencies at all levels face a dire shortage of workers, with roughly 44 percent considering leaving their jobs within the next five years. That’s why public health agencies should adopt intelligent automation tools.
Intelligent automation can significantly improve infectious disease reporting by automating the collection and transfer of relevant health information from EHRs. When a health worker records a particular symptom or disease case in a patient’s EHR, the system could automatically send the data directly to CDC, eliminating current administrative reporting burdens. Improvements in the EHR aren’t limited to public health use – intelligent automation systems can also enhance the care provided to patients and decision support provided to providers.
Intelligent automation systems could also scan and interpret lab reports and clinical notes to uncover disease cases that might otherwise elude health officials, and trigger reports to state and local authorities. Additionally, technology learns and adapts. Powered by artificial intelligence and machine learning, these systems can go beyond simple optical character recognition by leveraging natural language processing to understand context, reduce noise, and improve accuracy.
Conclusion
With a more modernized data infrastructure, public health leaders will be better equipped to identify and contain outbreaks, understand disease burdens, guide policy changes, evaluate and improve prevention and control strategies, and target research investments. The bottom line: enhanced data collection and analysis capabilities are critical to improving our nation’s public health outcomes.
About Kenyon Crowley
Kenyon Crowley, PhD is the Health Analytics Lead for Accenture Federal Services. Dr. Crowley brings nearly twenty years of health information technology expertise to his role. In his role at Accenture Federal Services, Dr. Crowley will help to accelerate the responsible and ethical use of AI and other advanced analytics tools across the federal health sector to help improve the well-being of all people in the country.