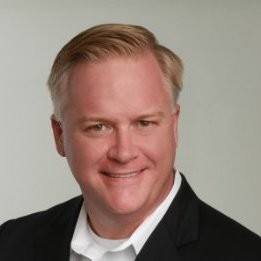
Since at least 2012, the world of medical records in both the public and private sectors remains dominated by a push toward interoperable data, and more broadly useful health data. Like everything else in American healthcare, the effort has been only moderately successful despite a price tag in the billions. In a modern digital world, where technologies in so many industries are bringing better insights and next generation efficiency – driven by cloud computing, big data, AI and machine learning – U.S. healthcare companies still operate without a single unified medical records format.
Worse still, we’re spending our money in all the wrong places, investing about $5 billion annually into initiatives in scheduling, monitoring and medication tracking. Yet far less is spent on access to data, or on interoperable medical records. Generations of medical records are sitting in countless VA hospitals warehouses, and in the databases of every major insurer, on the campuses of research universities and in the offices of every doctor large and small around the country. Within those records are medical outcomes for everyone who ever visited a doctor, the longitudinal data of broader populations, the hidden warning signs of future diseases, the untold answers to drug efficacy questions, the indicators and the contraindicators.
None of this data is broadly useful as presently constituted. And, none of it is ready for primetime consumption by the big data machine our nation is building elsewhere in industry and business – until now.
1. AI is Solving Medical Record Interoperability
Thanks to advances in AI and machine learning, a solution to the medical record interoperability question has emerged – and it’s the kind of advance that may drive the next generation of health data technology.
Cognitive technologies are being used more extensively than ever in health data to guide people through operating complex data processes, to consume the growing volume of health information and to extract actionable insights from the noise. There are numerous successful examples of applied AI in health data that are today delivering highly accurate information treatment and workforce augmentation.
One example of applied AI uses cognitive technologies to acquire, structure and deliver health records into research grade data sets. The process takes an enormous volume of paper medical records, and automates the extraction of information on each page, a capability which has helped break down the data illiquidity challenging the healthcare industry.
This is how it works: computer vision is used in coordination with natural language processing to convert millions of records and notes into electronic documents. The electronic records are then processed by real people, to disambiguate the information they contain and make adjustments to the information that was incorrectly transcribed by the system. Connect this process to a machine learning feedback loop, and we can continuously train the AI digitizing system with manual intervention for greater accuracy.
With this process, we can accumulate and refine our data sets until they reach a high level of accuracy, in the form of a structured digital record.
2. Imagine AI on a Healthcare Blockchain
Applying AI to records extraction is only a first step. Imagine the value AI could offer in the future to a potential healthcare blockchain, where the benefits of distributed records could be paired with cognitive technologies to target health insights automatically.
AI “agents” could one day soon be deployed on a health information blockchain to facilitate the discovery, development and delivery of an unprecedented level of personalized medicine.
If the blockchain were populated with health data, a swarm of AI agents — built and certified by leaders in the medical community — could search the records of individuals and populations in real-time, reflecting on each record as a single instance in a longitudinal databank. The AI agent could search for health-related warning signs and red flags that a doctor could easily miss in person and could aggregate the trends and insights of giant populations, predicting diseases in individuals, and outbreaks and overlooked cures for populations.
3. Better Data, Improved Insights
Whether we discuss the short-term victory already in action today, where we are creating digital and more sharable records, or the long-term vision, where our cognitive technologies power a generation of medicine-changing insights, one rule remains true. Accumulate enough structured records and the possibilities become endless.
If doctors have access to a patient’s entire medical history at the time of their visits, they become armed with far more meaningful information. If a research university could pull every cancer-related treatment outcome of the last five years with a few simple clicks, they would have far better statistical information at their fingertips as they search for the next generation of cures. Imagine, pharmaceutical companies selecting better trial groups, and pharmacists making better drug interaction choices; insurers improving claims and government health organizations more easily monitoring public health emergencies, catching correlations quicker and delivering information back to healthcare providers based on near real-time information.
Next-gen Tech to Solve Healthcare Challenges
Healthcare is under fire in America. Perhaps for good reason. The industry has for the most part not benefitted from a world growing more and more intelligent and efficient with each technological advance. Today, the industry should feel a sense of pressure to find new and better ways to share information and uncover patterns. With the sticker price for American healthcare being what it is, the attitudes of the general public and in the near enough future, the public policies of the country will increasingly demand either lower costs or better outcomes.
At its core, AI and machine learning may today be used to automate the digitization of medical records, but one day soon, the same tools could be the silver bullet. The tools exist today to create once-in-a-generation innovation, pushing U.S. health care into the modern digital era while simultaneously driving more actionable insight with less waste, more breakthroughs, improved care and better medical outcomes.