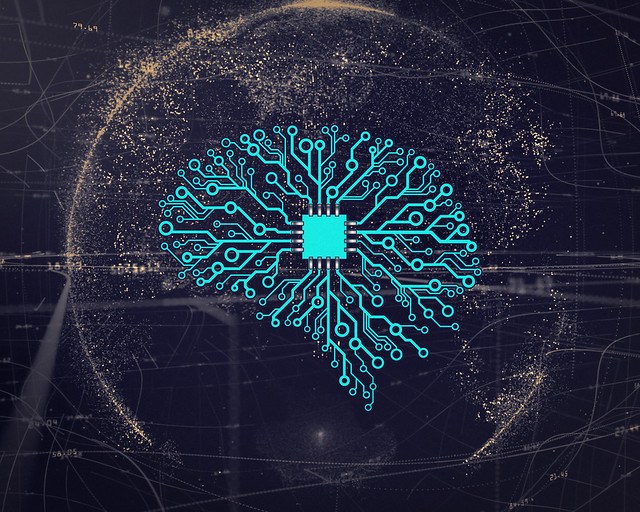
What You Should Know:
– Over the past few years, Artificial Intelligence (AI), and more specifically, Machine Learning (ML) technology, have experienced rapid adoption in the healthcare space as tools for diagnosis and decision-making. Such tools are intended to address challenges in the healthcare system to both processes and put into practice the proliferating medical findings, and also to support delivery of the promise of personalized and precision medicine.
– Unintended bias in AI and AI-driven healthcare applications is an evolving topic that developers, reviewers, and experts are still learning to address effectively and consistently. The Good Machine Learning Practices Working Team of the AFDO/RAPS Healthcare Products Collaborative believes the topic of bias could benefit from standard taxonomy, consistent approaches to identification, and addressing any identified sources of bias.
Understanding Bias in Artificial Intelligence
This is the third paper from the GMLP Working Team. The team is an industry-led volunteer team that has come together to lift the use, adoption, and implementation of AI through the teams’ collaborative and innovative efforts.
“Unintended bias in AI-enabled systems is a challenge in the development of ML algorithms,” says Pat Baird, working team co-chair and Sr. Regulatory Specialist at Philips. “The whitepaper outlines a framework for bias opportunity detection, assessment, and mitigation of unintended bias that can be used by product developers.” Leveraging established and proven methods currently used for risk analysis in healthcare systems specifically for unintended bias should enable more robust management, adds Baird. The paper asserts that bias can occur at points in the data supply chain and product supply chain. While focused on product developers, the paper also discusses perspectives from regulatory reviewers or other stakeholders of these solutions.
Currently, medical devices that use AI-enabled algorithms utilize machine learning (ML) as a mechanism to “learn” during the algorithm development process. Being diligent about the identification and mitigation of unintended biases is important as a guard against bias impacting certain patient populations and resulting in inequities in healthcare delivery. Unless bias is circumvented, resulting reports of inequity may lower trust in the output of an AI-enabled medical device and create a barrier to the adoption of ML technology in healthcare.
Unintended bias in AI-enabled healthcare applications (including medical devices) will continue to be an evolving topic that developers, regulators, and experts must continue to address. This paper leverages existing risk management and related processes and proposes a framework for bias opportunity detection, assessment, and mitigation of unintended bias.
Here are the additional key take-aways from the paper regarding identifying and dealing with bias:
Initial Bias Analysis:
Identify the Intended Use of the System: The first step in mitigating bias in AI/ML-based systems is to define the intended use and indications for use of the system against which the impact of unintended bias can be measured. The intended use of a medical device is variously identified as the “use for which a product, process, or service is intended according to the specifications, instructions, and information provided by the manufacturer,” and “… should take into account information such as the intended medical indication, patient population, part of the body or type of tissue interacted with, user profile, use environment, and operating principle” while considering “reasonably foreseeable misuse.” For software systems, a more granular view of intended use and indications for use and an elaboration of the above definitions can be found in a technical report providing guidance for non-device regulated software. The description, however, applies across the spectrum of device and non-device systems. “
Identify Applicable Biases: Accordingly, a first step in determining the presence of unintended bias could be to identify known foreseeable sources and types of bias associated with the intended use of the system.
Estimate the Impact of Bias on Intended Use: The next step would be to evaluate the impact of unintended bias on the intended use of the system. This could be achieved by:
● Providing a subjective narrative for each bias source/type/sequence combination that details the positive, negative, or neutral impact of the application of unintended bias on the intended use of the system.
● If the bias source/type/sequence combination has an impact on safety (including data privacy), then ensure the combination is considered as part of safety risk analysis.
● Establishing a qualitative scale to categorize each bias source/type/sequence combination incorporating the following elements:
● Likelihood that the bias source/type/sequence combination will occur during real- world use of the system.
● Likelihood that there will be impact to the intended use of the system if the bias source/type/sequence combination occurs.
● Degree (and categorical type) of impact to the intended use of the system if the bias source/type/sequence combination occurs. (Note: A more granular view of this attribute may be provided if there are variable degrees of impact each with a separate likelihood for that impact, given that the combination occurs.
● Scoring based on the qualitative scale of positive, negative, or neutral impact of the bias source/type/sequence combination to the intended use of the system.
● Indicator of safety impact or no safety impact (including data privacy) of the bias source/type/sequence combination.
Evaluate Bias:
Determine Acceptability of Each Bias: Based on the impact estimation above, determine whether each unintended bias source/type/sequence combination is acceptable (requiring no further mitigation) or unacceptable (requiring mitigations be identified and implemented). Consider one of the following methods for this determination:
– If a subjective narrative was used for impact estimation, provide a further narrative rationalizing whether the unintended bias source/type/sequence combination is acceptable or unacceptable.
– If a qualitative or quantitative scale was used for impact estimation, use a series of decision matrices to make the acceptability determination
Mitigate Unintended Bias
Determine Mitigations: For the unintended bias source/type/sequence combinations considered unacceptable, determine (and document in the bias analysis) mitigations (e.g., changes to requirements, architecture, design, data, code/algorithms, and/or algorithm training) necessary to bring bias source/type/sequence combinations to an acceptable state. The goal of determining appropriate mitigations is to reduce the likelihood that the bias source/type/sequence combination will occur, reduce the likelihood the bias source/type/sequence combination will have a negative impact if it occurs, and/or reduce the level of impact of the bias source/type/sequence combination.
Implement/Verify Mitigations: Where mitigations have been identified, implement them in the system using controlled design change and configuration management, where applicable. Verify implemented mitigations through both static and dynamic means (e.g., requirement reviews, technical reviews, code reviews, static code analysis, unit testing, integration testing, functional system testing).
Determine Residual Impact of Bias on Intended Use: Using the methods defined above to estimate and evaluate bias, determine the residual impact of unintended bias to the intended use of the system after bias mitigation mechanisms have been implemented and verified.
Determine Potential Bias Arising from Mitigations: Perform an estimation and evaluation of potential new or changed unintended bias source/type/sequence combinations that may arise from the implementation of bias mitigations. Where applicable, identify, implement, and verify mitigations on these new or changed bias source/type/sequence combinations.
Determine Completeness of Mitigations: Review all bias mitigation activities to ensure that the impacts from all identified bias source/type/sequence combinations have been considered and that all bias mitigation activities are complete.
Evaluate Overall Bias:
Determine Overall Acceptability of Bias in the Systems: Taking into consideration the residual impacts of all bias source/type/sequence combinations, determine whether the overall residual impact of bias source/type/sequence combinations is acceptable or unacceptable. If the overall impact is considered unacceptable, consider identifying and implementing further bias mitigations, making changes to intended use, identifying and documenting an acceptable benefit vs. bias assessment (refer to next paragraph), or reconsidering whether to release the system in its current configuration.
Articulate Benefit vs. Bias: For unintended bias source/type/sequence combinations that remain unacceptable, gather and review data to determine if the benefits of the intended use of the system (e.g., technical, clinical, economic) outweigh the residual impact for this specific combination. Consider also making an overall benefit vs. bias determination at the system level. If the benefits do not outweigh the residual impact, consider further system design changes (including bias mitigations and/or changes to intended use), or reconsidering whether to release the system in its current configuration.
Disclose Residual Bias: Disclose known residual bias source/type/sequence combinations including their predicted impact on the intended use of the system to relevant internal and external stakeholders (e.g., company management, customers, users, regulatory authorities). This may be done via a formal report, release notes, instructions for use, or other applicable communication mechanisms. In support of transparency, clear disclosure of intended bias needs to be disclosed as well